Understanding the Role of Normal Distribution in Mathematical Finance
In the world of mathematical finance, the normal distribution is one of the foundational building blocks used to model financial variables and make sense of market behavior. Whether you’re pricing options, evaluating risk, or building predictive models, understanding the normal distribution can give you powerful insights into the financial landscape. Let's explore how the normal distribution is applied in finance and why it is so important.
What Is Normal Distribution?
The normal distribution, often referred to as the bell curve due to its characteristic shape, is a probability distribution that describes how values of a random variable are likely to be distributed. It is symmetric around its mean, with most of the data points clustering around the center, and fewer points occurring as we move away from the mean.
In finance, we often use normal distribution to model asset returns, because it is mathematically convenient and aligns well with historical data for many financial metrics over short periods. The distribution is characterized by two parameters: the mean (which represents the average return) and the standard deviation (which indicates the degree of variation or volatility).

Mathematical Proof of Normal Distribution Properties
To understand the role of normal distribution in finance, it is essential to grasp some of its mathematical properties.
The probability density function (PDF) of a normal distribution is given by:
f(x) = (1 / (σ√2π)) * e^(-(x - μ)^2 / 2σ^2)
Where:
- μ is the mean of the distribution.
- σ is the standard deviation.
- x is the random variable.
The PDF represents the likelihood of different outcomes and is characterized by the symmetric bell curve shape, with the peak at the mean μ. The area under the curve represents the total probability, which equals 1.
For example, using the empirical rule (or 68-95-99.7 rule), we know that for a normal distribution:
- Approximately 68% of the data lies within one standard deviation of the mean.
- About 95% lies within two standard deviations.
- Roughly 99.7% lies within three standard deviations.
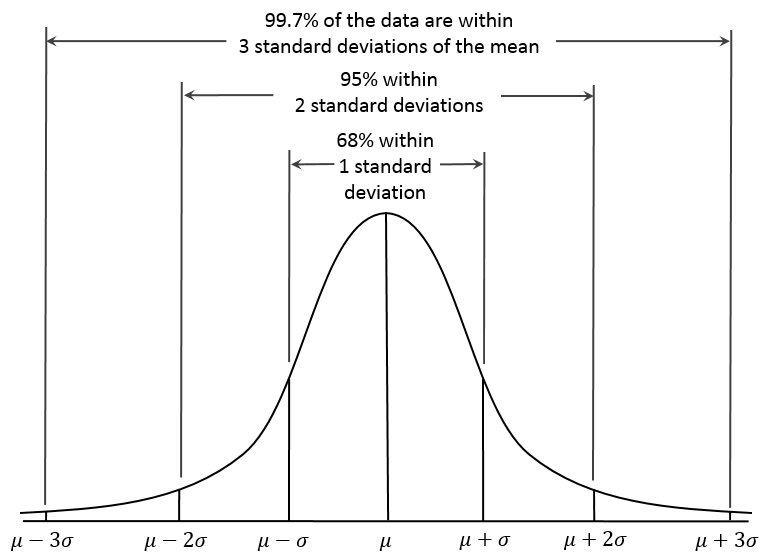
Applications in Mathematical Finance
1. Modeling Asset Returns
Financial analysts commonly assume that returns on assets, such as stocks or portfolios, follow a normal distribution, especially over short time horizons. This assumption allows for easier calculations of probabilities related to returns and risk. For example, by assuming normal distribution, we can estimate the probability that a given stock’s return will fall within a certain range over a period of time.
Example: Suppose the daily return of a stock is normally distributed with a mean of 0.1% and a standard deviation of 1%. We can calculate the probability that the return will fall between -1.9% and 2.1% by using the properties of the normal distribution, which gives us about a 95% confidence interval.
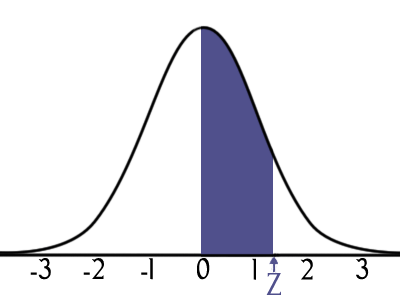
2. Calculating Value at Risk (VaR)
Value at Risk (VaR) is a risk management tool that quantifies the maximum expected loss over a specified time period at a given confidence level. The normal distribution plays a crucial role here, as it allows us to compute the likelihood of extreme losses by focusing on the distribution’s tail. Using the properties of the normal distribution, financial institutions can estimate potential losses and establish risk management strategies.
Mathematical Example: If the returns of a portfolio are normally distributed with a mean of 0.5% and a standard deviation of 2%, we can calculate the VaR at a 95% confidence level. Using the inverse of the cumulative distribution function (CDF), we find that the VaR is approximately -2.78%, indicating that there is a 5% chance that the portfolio will lose more than 2.78% in a given period.
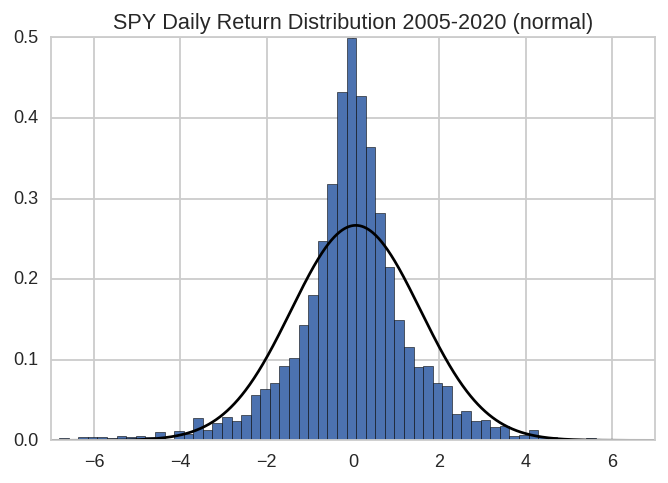
3. Black-Scholes Option Pricing Model
The Black-Scholes model, one of the most widely used tools for pricing options, relies on the assumption that the returns of the underlying asset follow a normal distribution. The model uses the concept of log-normality (which is closely related to normal distribution) to estimate the price of options. This is based on the idea that while asset prices cannot go below zero, their returns can still be symmetrically distributed around a mean.
Example: Suppose an investor wants to price a call option for a stock currently trading at $50, with a volatility (standard deviation) of 20%, a risk-free rate of 5%, and a time to maturity of 1 year. Using the Black-Scholes formula, which incorporates the assumption of normally distributed returns, the model will provide an estimate of the fair price of the option.
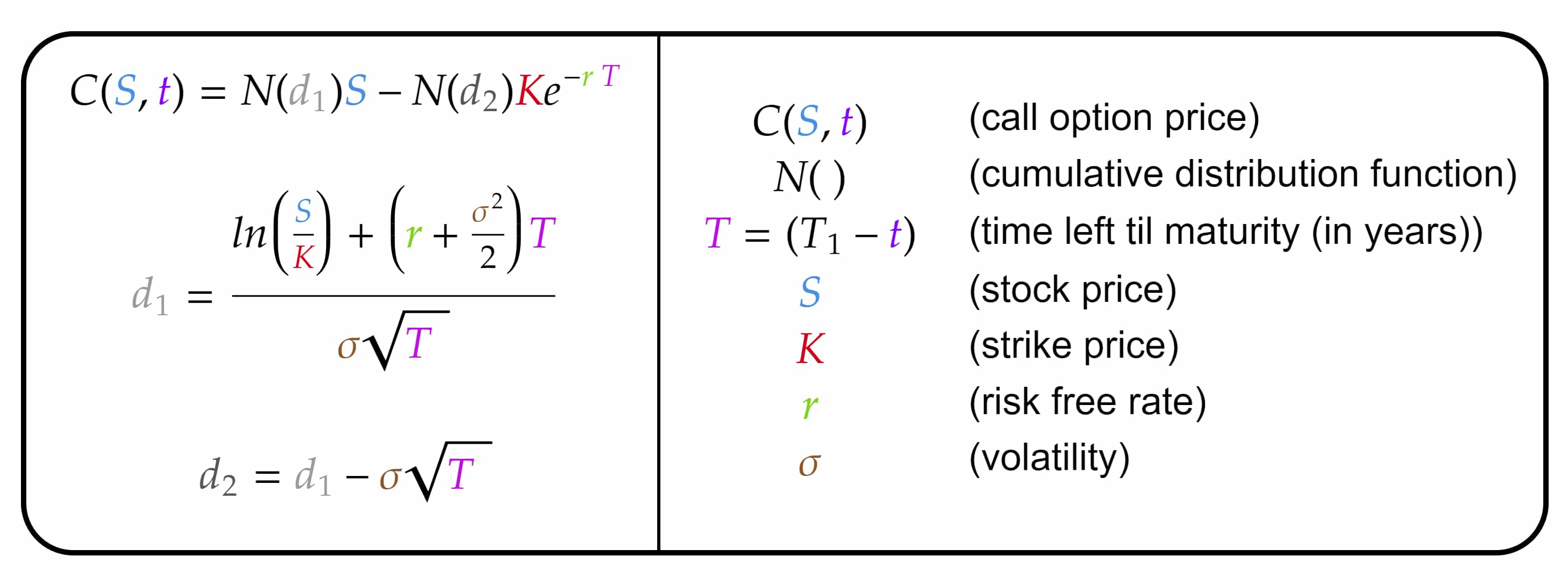
4. Portfolio Optimization
Harry Markowitz's Modern Portfolio Theory (MPT) also hinges on the assumption of normally distributed returns. Investors use the concept of normal distribution to model and quantify the risk and expected return of different asset combinations. By understanding how these returns are distributed, it becomes possible to build efficient portfolios that maximize return for a given level of risk.
Real-Life Example: Suppose an investor is deciding how to allocate capital between two assets, A and B. By assuming that the returns are normally distributed, the investor can use MPT to find the optimal weights that minimize risk while achieving a target return. The covariance between the assets, combined with their means and standard deviations, helps determine the efficient frontier, guiding the investor’s decision-making process.
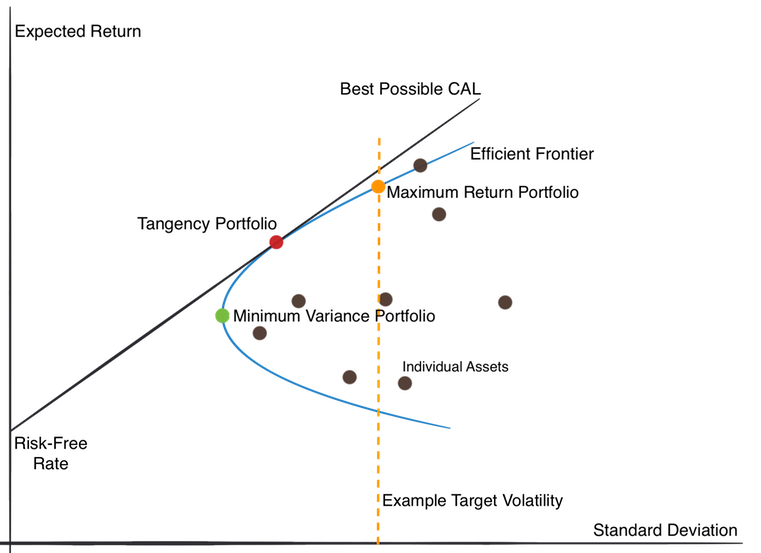
Limitations of Using Normal Distribution
While the normal distribution is powerful, it's not without limitations. Real financial returns often exhibit fat tails and skewness, meaning that extreme events (like market crashes) happen more frequently than what would be predicted by a perfect normal distribution. This phenomenon is why some models, like the Black-Scholes, may underestimate the probability of large market moves.
Additionally, the assumption of normal distribution does not account for market behavior driven by emotions or external shocks, which can lead to more erratic price movements. Thus, although the normal distribution is a useful starting point, analysts often use more advanced models, such as those incorporating leptokurtic or heavy-tailed distributions, to capture these nuances.
Conclusion
The normal distribution is a cornerstone of mathematical finance, enabling analysts to model returns, measure risk, and optimize portfolios with relative ease. Its convenient mathematical properties make it an essential tool, particularly in the areas of option pricing, risk management, and investment strategy. However, it’s crucial for practitioners to be mindful of its limitations and consider supplementary models when dealing with real-world financial data.
By appreciating both the strengths and weaknesses of the normal distribution, you can develop a more nuanced understanding of financial markets and make better-informed decisions in a complex environment.